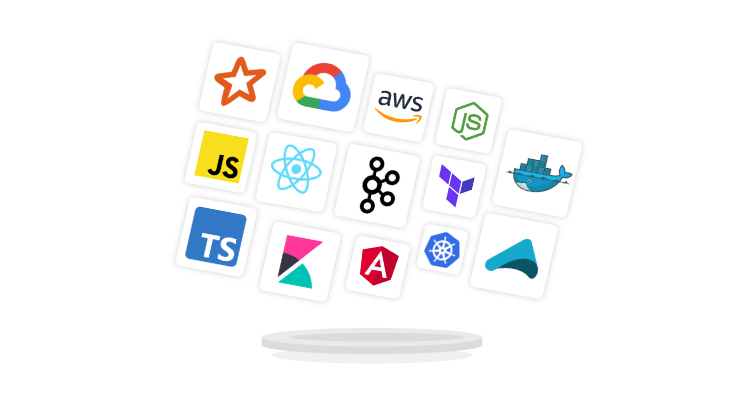
How to create a macro transforming a function
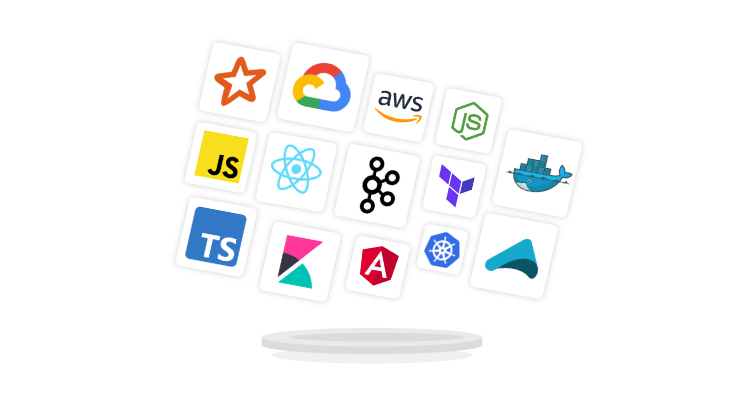
Hello! In this post we’ll go through a short introduction to macros and learn how to use them to our advantage.
I like maths, so we’ll create a simplified derivative macro that will replace expressions like:
https://gist.github.com/pjazdzewski1990/81086b50f3c3befaff82
with
https://gist.github.com/pjazdzewski1990/f359d4e266b7bd0a5fbf
Don’t worry if you aren’t familiar with calculus and derivatives: I’ll provide a simplified explanation later, and you’ll learn quite a lot nevertheless.
So what do we need? We need to analyze the function’s method calls – in this example, we’ll split the function’s body into a sum of expressions that we can differentiate.
The complete implementation of the derivative macro, as well as the hello macro, is available on the master
branch of our GitHub repo.
Let’s get started!
What is a macro, actually?
A macro is generally a special function – that is, special in that it’s run at compile time. It has access to its arguments not by value (or by name), but as abstract syntax trees with information about their types. I’ll explain what that means in a while.
Some macros can even transform whole classes – they are called annotation macros. For example, they can read class fields and generate a JSON conversion from that.
However, annotation macros are beyond the scope of this post – we’re only going to focus on def macros, which are macros that look like functions.
For now, let’s start actually getting our macros to work.
Configuration
If you want to use macros in your library, you’ll need to add "org.scala-lang" % "scala-reflect" % "2.11.7"
to your dependencies in build.sbt
. The clients of your library won’t be required to do that, though.
Keep in mind that macros have to be compiled before their usages – which means you won’t be able to even test your macros in the same compilation run. To use your macros, you can try one of the following:
sbt console
– your macros will be compiled and usable- Put your macros in one sbt subproject and use them in another one
- Include your macro project as an external project dependency in your IDE
- Build your macro project and include it as a jar or sbt/maven/gradle dependency
Having added the scala-reflect
dependency, we can start working on our first macro.
Hello world macro
We’ll create a new object
, namely Macros
for that purpose – you can use any name for it.
https://gist.github.com/pjazdzewski1990/8e6214a437f4a01ba1a9
Note the import – we imported the blackbox
version – there’s also a whitebox
one. The main difference between them is that white-box macros can have a more concrete return type than they define. If you’re interested in the full definition, check out Blackbox Vs Whitebox by Eugene Burmako. I’ll use black-box macros in this post just because they faithfully follow their type signatures.
The first macro we’ll add is a “hello world” one – so we’ll want expressions like hello
to be replaced with println("hello!")
in compile time. I’ll write the implementation first, then I’ll explain it part by part.
In object Macros
:
https://gist.github.com/pjazdzewski1990/d823a7d9f257926f9e01
A lot of things happening there – so let me explain:
In the first line, we say that hello
invokes a macro expansion, which, in this case, is done by helloImpl
. We’ve also added a return type annotation to the hello
method – with Scala 2.12, the return type of macros without return type annotations will be inferred as Unit
(which is not a problem in this case, but will become one when we start working with non-Unit
macros).
helloImpl
’s first (and only, for the time being) parameter list consists only of a blackbox.Context
, and the whole function declares its return type as c.Expr[Unit]
. This means that when the macro is expanded, the result will be an expression of the type Unit
.
Let’s take a look at helloImpl
’s body now – it starts with an import – and you’re going to see that import in basically every macro you encounter, as it brings a lot of useful functions and types to the scope. In this case, we’re using the q
function, which is a string interpolator used for creating c.Tree
s – you can insert any expression in the so-called quasiquotes and it’ll be compiled into a proper tree – I hope that makes sense.
There are a few more ways to do what we did in the last line in the function body. We could use reify
(a macro imported from c.universe), which turns a Scala expression into a c.Expr
:
https://gist.github.com/pjazdzewski1990/69fad0393cf6c86f859a
This actually looks pretty simple, but will become more complicated when we add additional arguments to our macro.
Or we could construct the tree manually, which is the old-fashioned way – it was more commonly used before quasiquotes were introduced:
https://gist.github.com/pjazdzewski1990/a0742ca3b45dd16817de
I’ll explain the Apply(...)
part later as well.
Now we have a macro that we can use in our Scala code:
https://gist.github.com/pjazdzewski1990/94711acfbc6954d1175e
You may be wondering – what’s the advantage of the macro we’ve written over a function that calls println
the usual way? Imagine we wrote the whole thing as follows:
https://gist.github.com/pjazdzewski1990/980478129280425846af
This way, we have a simpler implementation. However, this way, we lose the advantages of a macro. If hello
had arguments, we could only access their values (and we’ll need more than that to complete the differentiation task later). We also have a nested function call, so every use of hello
will involve additional runtime overhead related to adding a frame to the call stack.
On the other hand, when we call macro helloImpl
, it’s replaced by the expanded result of helloImpl
in compile-time. This way, we avoid this little bit of runtime overhead related to an additional call of helloImpl
inside hello
. While it doesn’t make that much of a difference here, there are more advanced use cases where macros show their power – and we’ll see them in a couple of moments.
What if we wanted to call our function like hello("world")
? How much more complex would the macro become? Let’s see.
Adding an argument to a macro
The updated hello macro definition will look like this:
https://gist.github.com/pjazdzewski1990/2d06e22bb5118b85be14
And the new macro implementation, using quasiquotes:
https://gist.github.com/pjazdzewski1990/12d078f075f00765cbd1
Not a lot more complicated, it turns out. The only things that have changed are:
- an additional
s: c.Expr[String]
argument, in an argument list separate from the context one. Note that the name of the argument (s
) must be the same as in thehello2
function. - We’ve added an interpolation of
s.tree
– this shows us that to get an expression’s tree, you call thetree
method on it.
Now we can use our macro:
https://gist.github.com/pjazdzewski1990/5848c887a3920a2e51ee
What about the non-quasiquote versions? Turns out, if we want to use reify
, we’ll need to do it like this:
https://gist.github.com/pjazdzewski1990/90c5f13d7e61d8fce76d
In order to use an c.Expr[T]
inside a reify
block, you have to call splice
on it – which I did. The method’s return type is T
– but you can’t use this method anywhere outside reify
, as it only serves for a mark that reify
understands when it embeds the Expr
’s tree into its result.
According to the docs of reify, reify { expr.splice.foo }
is equivalent to the following AST:
https://gist.github.com/pjazdzewski1990/9815c748cc530f944a5d
Let’s see what the tree-based implementation looks like.
https://gist.github.com/pjazdzewski1990/2e46d8d3e1eecad93ca6
Oh boy – that’s not so pretty, is it? It’s probably time to take a look at what the tree actually represents.
Before we start: how did I get this tree representation?
https://gist.github.com/pjazdzewski1990/18d1a56afe4aa1f25034
You can use the show
method, but it acts the same as a Tree
’s toString
, so it doesn’t yield the complete tree representation.
Understanding Trees
The tree starts with an Apply
, so let’s take a look at that.
https://gist.github.com/pjazdzewski1990/526d5b5f6730e2f6b4c5
Apply
is basically an application of function fun
with arguments args
. In this case, the function is Ident(TermName("println"))
, which is just another way to say that we are looking for a value/def called “println” in context c
.
Let’s go on to this Apply’s parameter list – it has one child, namely another Apply
, whose function is a Select
, and its argument is a Literal(Constant("!"))
– which, similarly to a value/def reference, is basically a compile-time constant reference – in this case, a String
containing an exclamation mark.
What is a Select
, now?
https://gist.github.com/pjazdzewski1990/8a3217a191ca96839b5c
Select(qualifier, name)
is an AST node that corresponds to qualifier.name
. Let me recall how it was used in our example.
https://gist.github.com/pjazdzewski1990/be80a8f10c6727de548f
The inner Select
in this snippet corresponds to "hello ".$plus
, which is a function, later applied with s
as its argument. The result of that function call is then used as a qualifier
in the external Select
, then a $plus
selection on that qualifier is made.
What does the whole AST correspond to, now?
https://gist.github.com/pjazdzewski1990/24d08f5aa03cb876fc11
Which is basically the same as
https://gist.github.com/pjazdzewski1990/11cedbc01340a3b21687
So… that’s exactly what we wanted! Great success.
Pattern mathing trees
Turns out, you can use pattern matching basically the same way you would construct a Tree yourself. In this section, I won’t use
https://gist.github.com/pjazdzewski1990/d2ffc71f2ef5af0f8cc9
but
https://gist.github.com/pjazdzewski1990/e4c43199d694006d5d79
this way I’ll be able to use macros’ features directly inside the REPL, without creating a def macro.
https://gist.github.com/pjazdzewski1990/1361f387ec831bd896ce
And, using quasiquotes.
https://gist.github.com/pjazdzewski1990/2468980777913a337681
You can pattern match more precisely by using the AST syntax inside the quasiquote matcher:
https://gist.github.com/pjazdzewski1990/10d0aa48e71e7b9ab3ab
You can also split the match in two, to make it more readable.
Let’s get back to our problem defined in the beginning of this post.
Case study: differentiation
For those that aren’t familiar with calculus, a quick explanation:
A derivative of function f(x)
with respect to its variable x
can be roughly expressed as the speed of f(x)
’s change relative to x
’s change. It’s a function as well, and it has a value for every x
in its domain. For a more complete definition, consult the Wikipedia article on derivatives.
A simple example: if f(x) = 3x + 5
, and x
changes by 1
, f(x)
changes by 3
. See it for yourself on the plot of 3x+5
for 0 ≤ x ≤ 1.
To find the derivative of our input, the steps we have to follow are roughly:
- Extract expressions that are being added / substracted in the function body
- Transform them into components that we can convert to their derivatives’ trees later
- Convert the components into their derivatives
What expressions do we want to find derivatives of? We’ll start by creating an MVP macro that can understand the three simplest kinds of expressions:
Function (f(x)) | Derivative (f’(x)) |
---|---|
x | 1 |
a (real number) | 0 |
g(x) + h(x) | g’(x) + h’(x) |
Our testing function will be:
https://gist.github.com/pjazdzewski1990/755e4a18cc3053b8db36
Okay. According to the steps mentioned earlier, we can estimate what our new macro will look like (I used a new singleton object for clarity):
https://gist.github.com/pjazdzewski1990/49feded230909c07deb5
Take note that extractComponents
and toTree
have an implicit
Context
parameter in their declarations – they’ll be useful later.
In this snippet, I defined a Component
trait with a toTree
method and a derive
method. It will be a common base for classes representing mathematical expressions our macro will be able to understand and differentiate (like a variable, a numeric constant, a multiplication etc.).
Our new object has 3 functions:
derivative
– which points to the macro functionderivativeImpl
derivativeImpl
– which transforms anExpr[Double => Double]
into another expression of the same type.extractComponents
– which takes an AST and extractsComponent
s from that AST
First, let’s try and implement the actual macro implementation:
https://gist.github.com/pjazdzewski1990/aa665c41d8cde5fb0e16
A new thing that’s appeared is a Function
extractor. We’re using it to extract the function’s argument and body this way:
https://gist.github.com/pjazdzewski1990/0745d6e2373d3e1c5b39
Which is basically the same as:
https://gist.github.com/pjazdzewski1990/827b074c5fbd893ac855
Unfortunately, quasiquotes aren’t precise enough to enable extracting the parameter’s name. Thus, in order not to mix styles in this section, I used AST pattern matching.
The Function
extractor takes as arguments a List
of ValDef
s (the function arguments), and the function body’s Tree
. So, in our case, we’re using this extractor to get the function argument’s name: TermName
and funcBody: Tree
out of our function definition expression f
’s tree.
Let’s take a look at the other lines in derivativeImpl
:
https://gist.github.com/pjazdzewski1990/1ab6f35507ce99e9e974
These should be self-explanatory – we’re running extractComponents with our function’s body tree as the argument, additionally passing the Context
(because it can’t be passed implicitly in this… “context”).
Later, we’re mapping the components to their appropriate derivatives and summing them using quasiquotes (yes, you can interpolate trees inside a quasiquote). Then, the result of that reduction, which is our new function body, is inserted into the resulting function, wrapped in an expression.
Now, we need to implement extractComponents
:
https://gist.github.com/pjazdzewski1990/c7741ccb7f4308f831cf
We have two cases for the tree – it’s either an addition, or a single component – we don’t support substraction yet.
You may be wondering – how will a function with more than two components fit into the first branch of the match, if it only cares about two?
Let’s recall what operators in Scala are – they are method calls. So, an expression like x + 5 + x
is actually desugarized into a chain of calls: x.+(5).+(x)
– and indeed, if we display a
and b
’s trees, we’ll see:
https://gist.github.com/pjazdzewski1990/529bf1241aa5a9a724a9
Which means our match worked – but we’ll still have to divide a
into components. Let’s update our match
, renaming a
and b
to nextTree
and arg
respectively:
https://gist.github.com/pjazdzewski1990/72660a82b8398e8dc0a5
But what should we do with arg
? We might use recursion again, and join the results with :::
, but it’ll be simpler to do the same thing we’ll do in the second branch of the match
statement – which is… well, we’ll need another function for that. Let’s call it getComponent
. Now our match statement will look like this:
https://gist.github.com/pjazdzewski1990/4e98a788d58045328dfe
Seems about right, doesn’t it? Now we need to implement getComponent
:
https://gist.github.com/pjazdzewski1990/5b58cf5c841c972fdaa6
Let’s go back to our MVP idea – we want to be able to find a derivative of a sum of x
s and real numbers, and we want them to be represented by Component
subclass instances. Let’s define these subclasses.
https://gist.github.com/pjazdzewski1990/52fd6dba982f12f86ffb
As I mentioned in the earlier part of this post, a val
’s AST representation is Ident(TermName(x))
, where x
is the val
’s name. Similarly, a constant literal’s AST representation is Literal(Constant(x))
, where x
is the constant’s value. We can apply this knowledge and implement toTree
in both Component
implementations:
https://gist.github.com/pjazdzewski1990/b045ad61ab1fd951a6eb
Now we need to make getComponent
know how it can extract a Component
from a tree. We can use the same AST patterns as in the toTree
implementations for that:
https://gist.github.com/pjazdzewski1990/6a667928f22f4589c50b
The last thing we need to do is implement def derive: Component
in our Component
classes. Following the derivative table above, we can do it very easily:
https://gist.github.com/pjazdzewski1990/7820698b54d4fa521569
Turns out, we’ve finished our MVP! You can see its whole implementation in the mvp
branch of the GitHub repository accompanying this post, which you’ll find in the links section at the end of this post.
Let’s run our macro with our test function.
https://gist.github.com/pjazdzewski1990/f28f19d8a5d5cff96ec3
According to the table of derivatives, we expect the derivative of:
https://gist.github.com/pjazdzewski1990/f93cf1f73d457e7fe99b
to be
https://gist.github.com/pjazdzewski1990/16e4e7b5690d4390a932
So, for every argument x
, our derivative will be equal to 2. Let’s see (for a modest amount of x
s).
https://gist.github.com/pjazdzewski1990/7d4a1631374433629f40
Success! Our MVP is working. Now we can add a few more expressions that we can differentiate.
More advanced differentiation
What’s new on our plate?
Function (f(x)) | Derivative (f’(x)) |
---|---|
x | 1 |
a (real number) | 0 |
g(x) + h(x) | g’(x) + h’(x) |
g(x) * h(x) | g(x) * h’(x) + g’(x) * h(x) |
pow(x, n) | n * pow(x, n-1) |
The g(x) * h(x)
rule also applies to expressions like n*x
– and their derivatives can be simplified as n
, but for this project, which is only proof of concept, we’ll omit that special case – it’ll work anyways, but the resulting AST will be just a bit more complex.
We’ll start by adding missing Component
classes.
https://gist.github.com/pjazdzewski1990/620eccfd40c601dfd45e
This one will take care of negated components in functions like f(x) = -x + ...
. Corresponding getComponent
case:
https://gist.github.com/pjazdzewski1990/3f621e392fe71fa32027
As we are here, we can support the unary plus operator as well.
https://gist.github.com/pjazdzewski1990/5a8c82d0bc5a982e8e86
And now that we can negate components, let’s make sure functions with substractions are working. In extractComponents
, add:
https://gist.github.com/pjazdzewski1990/dacea674513ba3c16777
Just out of curiosity, let’s see what q"(x: Double) => -x"
’s body would look like as an AST.
https://gist.github.com/pjazdzewski1990/02f695cb62214693e36a
What’s interesting is that there’s no Apply when we’re looking at unary operators. Anyways, back to our missing components.
You’ve previously seen that we support expressions in the form of a sum… but what if they are nested inside a multiplication, like 2 * (x + 5)
? Turns out, we need to add a Component
for that to make sure such expressions work once we take care of multiplication.
https://gist.github.com/pjazdzewski1990/b828fe32542128f7cf66
appropriate getComponent
case:
https://gist.github.com/pjazdzewski1990/c988929da5808d02c57a
And a substraction case:
https://gist.github.com/pjazdzewski1990/b6f5c25ad2f6ad5dad59
One of the last components we need to add is multiplication.
https://gist.github.com/pjazdzewski1990/15b18054f67cf965e6b7
Corresponding getComponent
case:
https://gist.github.com/pjazdzewski1990/bd9271bc8c9064395dc0
Adding component types is getting pretty predictive, isn’t it? We could make it more complex by adding a pattern match in derive
, checking whether one of the factors of the multiplication is a DoubleConstant
, and the other a Variable
– if they were, we could just return the constant as the derivative – but as I said before, this is just a proof of concept, so we’ll omit that.
The last component we’ll need to satisfy our original needs are powers of x. One thing that we need to remember now is that functions like Math.pow(2, x)
are beyond the scope of our case study – so we’ll require powers to have x as the base (Math.pow(x, n)
) – but only in the derive
function, and not in the class’s constructor arguments (so we can add the missing implementation later without much hassle).
Also, we might add a special case for when the exponent is 2 (then we could omit the exponentation in the derivative) or 1 (so the result would be 1) – but that will only make our implementation more complicated, so we won’t do that in this post.
https://gist.github.com/pjazdzewski1990/90a4ddf9e79902e6b0b7
And finally, the getComponent
case:
https://gist.github.com/pjazdzewski1990/fb9c0d89ba6941af7bba
The this
has to be there – it seems to be related to the fact that java.lang._
is a default import in Scala. Without it, our match would fail, unless we only wanted to understand more verbose calls like java.lang.Math.pow($a, $b)
.
And we’re done! You can use our derivative macro with a complicated function using all components:
https://gist.github.com/pjazdzewski1990/17935be454bd8ee7bd85
The function we supplied can be simplified as
https://gist.github.com/pjazdzewski1990/3dbd4925888afdb4a503
And its derivative is f'(x) = 8 * x + 6
If our implementation is correct, the resulting derivative will be equal to the above for every x we can imagine. Let’s test that for -1000 ≤ x ≤ 1000, stepping by 0.1.
https://gist.github.com/pjazdzewski1990/00d1af1ee16c9cb305de
Summary
As you can see, it works! We’ve successfully implemented a quite complex macro. We’ve learned what Scala’s ASTs look like, how we can extract nodes from them, and create new trees. I hope I gave you a good understanding of def macros and that you’ve learned how to use them in your own code.
If you have any questions regarding this post, or maybe def macros in general, please comment on this post, and I’ll do my best to answer.
Thanks for reading!
Links
The “hello world” macro was based on Adam Warski’s implementation.
You can find the source code for this post on our GitHub.
Other articles that helped me when writing this post were:
- Scala documentation post by Eugene
- Another one about symbols, ASTs and types
- Scala Unless-when via Macros by Lloyd Chan
Do you like this post? Want to stay updated? Follow us on Twitter or subscribe to our Feed.
See also